How to address limitations of naive RAG pipelines by implementing targeted advanced RAG techniques in Python
Difference between Naive and Advanced RAG (Image by the author, inspired by [1])
A recent survey on Retrieval-Augmented Generation (RAG) [1] summarized three recently evolved paradigms:
The advanced RAG paradigm comprises of a set of techniques targeted at addressing known limitations of naive RAG. This article first discusses these techniques, which can be categorized into pre-retrieval, retrieval, and post-retrieval optimizations.
In the second half, you will learn how to implement a naive RAG pipeline using Llamaindex in Python, which will then be enhanced to an advanced RAG pipeline with a selection of the following advanced RAG techniques:
This article focuses on the advanced RAG paradigm and its implementation. If you are unfamiliar with the fundamentals of RAG, you can catch up on it here:
Retrieval-Augmented Generation (RAG): From Theory to LangChain Implementation
What is Advanced RAG
With the recent advancements in the RAG domain, advanced RAG has evolved as a new paradigm with targeted enhancements to address some of the limitations of the naive RAG paradigm. As summarized in a recent survey [1], advanced RAG techniques can be categorized into pre-retrieval, retrieval, and post-retrieval optimizations.
Difference between Naive and Advanced RAG (Image by the author, inspired by [1])
Pre-retrieval optimization
Pre-retrieval optimizations focus on data indexing optimizations as well as query optimizations. Data indexing optimization techniques aim to store the data in a way that helps you improve retrieval efficiency, such as [1]:
Sentence window retrieval
Additionally, pre-retrieval techniques aren’t limited to data indexing and can cover techniques at inference time, such as query routing, query rewriting, and query expansion.
Retrieval optimization
The retrieval stage aims to identify the most relevant context. Usually, the retrieval is based on vector search, which calculates the semantic similarity between the query and the indexed data. Thus, the majority of retrieval optimization techniques revolve around the embedding models [1]:
There are also other retrieval techniques besides vector search, such as hybrid search, which often refers to the concept of combining vector search with keyword-based search. This retrieval technique is beneficial if your retrieval requires exact keyword matches.
Improving Retrieval Performance in RAG Pipelines with Hybrid Search
Post-retrieval optimization
Additional processing of the retrieved context can help address issues such as exceeding the context window limit or introducing noise, thus hindering the focus on crucial information. Post-retrieval optimization techniques summarized in the RAG survey [1] are:
Re-ranking
For additional ideas on how to improve the performance of your RAG pipeline to make it production-ready, continue reading here:
A Guide on 12 Tuning Strategies for Production-Ready RAG Applications
Prerequisites
This section discusses the required packages and API keys to follow along in this article.
Required Packages
This article will guide you through implementing a naive and an advanced RAG pipeline using LlamaIndex in Python.
pip install llama-index
In this article, we will be using LlamaIndex v0.10. If you are upgrading from an older LlamaIndex version, you need to run the following commands to install and run LlamaIndex properly:
pip uninstall llama-index
pip install llama-index --upgrade --no-cache-dir --force-reinstall
LlamaIndex offers an option to store vector embeddings locally in JSON files for persistent storage, which is great for quickly prototyping an idea. However, we will use a vector database for persistent storage since advanced RAG techniques aim for production-ready applications.
Since we will need metadata storage and hybrid search capabilities in addition to storing the vector embeddings, we will use the open source vector database Weaviate (v3.26.2), which supports these features.
pip install weaviate-client llama-index-vector-stores-weaviate
API Keys
We will be using Weaviate embedded, which you can use for free without registering for an API key. However, this tutorial uses an embedding model and LLM from OpenAI, for which you will need an OpenAI API key. To obtain one, you need an OpenAI account and then “Create new secret key” under API keys.
Next, create a local .env file in your root directory and define your API keys in it:
OPENAI_API_KEY="<YOUR_OPENAI_API_KEY>"
Afterwards, you can load your API keys with the following code:
# !pip install python-dotenv
import os
from dotenv import load_dotenv,find_dotenv
load_dotenv(find_dotenv())
Implementing Naive RAG with LlamaIndex
This section discusses how to implement a naive RAG pipeline using LlamaIndex. You can find the entire naive RAG pipeline in this Jupyter Notebook. For the implementation using LangChain, you can continue in this article (naive RAG pipeline using LangChain).
Step 1: Define the embedding model and LLM
First, you can define an embedding model and LLM in a global settings object. Doing this means you don’t have to specify the models explicitly in the code again.
from llama_index.embeddings.openai import OpenAIEmbedding
from llama_index.llms.openai import OpenAI
from llama_index.core.settings import Settings
Settings.llm = OpenAI(model="gpt-3.5-turbo", temperature=0.1)
Settings.embed_model = OpenAIEmbedding()
Step 2: Load data
Next, you will create a local directory named data in your root directory and download some example data from the LlamaIndex GitHub repository (MIT license).
!mkdir -p 'data'
!wget '<https://raw.githubusercontent.com/r...amples/data/paul_graham/paul_graham_essay.txt>' -O 'data/paul_graham_essay.txt'
Afterward, you can load the data for further processing:
from llama_index.core import SimpleDirectoryReader
# Load data
documents = SimpleDirectoryReader(
input_files=["./data/paul_graham_essay.txt"]
).load_data()
Step 3: Chunk documents into nodes
As the entire document is too large to fit into the context window of the LLM, you will need to partition it into smaller text chunks, which are called Nodes in LlamaIndex. You can parse the loaded documents into nodes using the SimpleNodeParser with a defined chunk size of 1024.
from llama_index.core.node_parser import SimpleNodeParser
node_parser = SimpleNodeParser.from_defaults(chunk_size=1024)
# Extract nodes from documents
nodes = node_parser.get_nodes_from_documents(documents)
Step 4: Build index
Next, you will build the index that stores all the external knowledge in Weaviate, an open source vector database.
First, you will need to connect to a Weaviate instance. In this case, we’re using Weaviate Embedded, which allows you to experiment in Notebooks for free without an API key. For a production-ready solution, deploying Weaviate yourself, e.g., via Docker or utilizing a managed service, is recommended.
import weaviate
# Connect to your Weaviate instance
client = weaviate.Client(
embedded_options=weaviate.embedded.EmbeddedOptions(),
)
Next, you will build a VectorStoreIndex from the Weaviate client to store your data in and interact with.
from llama_index.core import VectorStoreIndex, StorageContext
from llama_index.vector_stores.weaviate import WeaviateVectorStore
index_name = "MyExternalContext"
# Construct vector store
vector_store = WeaviateVectorStore(
weaviate_client = client,
index_name = index_name
)
# Set up the storage for the embeddings
storage_context = StorageContext.from_defaults(vector_store=vector_store)
# Setup the index
# build VectorStoreIndex that takes care of chunking documents
# and encoding chunks to embeddings for future retrieval
index = VectorStoreIndex(
nodes,
storage_context = storage_context,
)
Step 5: Setup query engine
Lastly, you will set up the index as the query engine.
# The QueryEngine class is equipped with the generator
# and facilitates the retrieval and generation steps
query_engine = index.as_query_engine()
Step 6: Run a naive RAG query on your data
Now, you can run a naive RAG query on your data, as shown below:
# Run your naive RAG query
response = query_engine.query(
"What happened at Interleaf?"
)
Implementing Advanced RAG with LlamaIndex
In this section, we will cover some simple adjustments you can make to turn the above naive RAG pipeline into an advanced one. This walkthrough will cover the following selection of advanced RAG techniques:
As we will only cover the modifications here, you can find the full end-to-end advanced RAG pipeline in this Jupyter Notebook.
Indexing optimization example: Sentence window retrieval
For the sentence window retrieval technique, you need to make two adjustments: First, you must adjust how you store and post-process your data. Instead of the SimpleNodeParser, we will use the SentenceWindowNodeParser.
from llama_index.core.node_parser import SentenceWindowNodeParser
# create the sentence window node parser w/ default settings
node_parser = SentenceWindowNodeParser.from_defaults(
window_size=3,
window_metadata_key="window",
original_text_metadata_key="original_text",
)
The SentenceWindowNodeParser does two things:
During retrieval, the sentence that most closely matches the query is returned. After retrieval, you need to replace the sentence with the entire window from the metadata by defining a MetadataReplacementPostProcessor and using it in the list of node_postprocessors.
from llama_index.core.postprocessor import MetadataReplacementPostProcessor
# The target key defaults to `window` to match the node_parser's default
postproc = MetadataReplacementPostProcessor(
target_metadata_key="window"
)
...
query_engine = index.as_query_engine(
node_postprocessors = [postproc],
)
Retrieval optimization example: Hybrid search
Implementing a hybrid search in LlamaIndex is as easy as two parameter changes to the query_engine if the underlying vector database supports hybrid search queries. The alpha parameter specifies the weighting between vector search and keyword-based search, where alpha=0 means keyword-based search and alpha=1 means pure vector search.
query_engine = index.as_query_engine(
...,
vector_store_query_mode="hybrid",
alpha=0.5,
...
)
Post-retrieval optimization example: Re-ranking
Adding a reranker to your advanced RAG pipeline only takes three simple steps:
# !pip install torch sentence-transformers
from llama_index.core.postprocessor import SentenceTransformerRerank
# Define reranker model
rerank = SentenceTransformerRerank(
top_n = 2,
model = "BAAI/bge-reranker-base"
)
...
# Add reranker to query engine
query_engine = index.as_query_engine(
similarity_top_k = 6,
...,
node_postprocessors = [rerank],
...,
)
There are many more different techniques within the advanced RAG paradigm. If you are interested in further implementations, I recommend the following two resources:
Summary
This article covered the concept of advanced RAG, which covers a set of techniques to address the limitations of the naive RAG paradigm. After an overview of advanced RAG techniques, which can be categorized into pre-retrieval, retrieval, and post-retrieval techniques, this article implemented a naive and advanced RAG pipeline using LlamaIndex for orchestration.
The RAG pipeline components were language models from OpenAI, a reranker model from BAAI hosted on Hugging Face, and a Weaviate vector database.
We implemented the following selection of techniques using LlamaIndex in Python:
You can find the Jupyter Notebooks containing the full end-to-end pipelines here:
Enjoyed This Story?
Subscribe for free to get notified when I publish a new story.
Get an email whenever Leonie Monigatti publishes.
Find me on LinkedIn, Twitter, and Kaggle!
Disclaimer
I am a Developer Advocate at Weaviate at the time of this writing.
References
Literature
[1] Gao, Y., Xiong, Y., Gao, X., Jia, K., Pan, J., Bi, Y., … & Wang, H. (2023). Retrieval-augmented generation for large language models: A survey. arXiv preprint arXiv:2312.10997.
Images
If not otherwise stated, all images are created by the author.
Advanced Retrieval-Augmented Generation: From Theory to LlamaIndex Implementation was originally published in Towards Data Science on Medium, where people are continuing the conversation by highlighting and responding to this story.
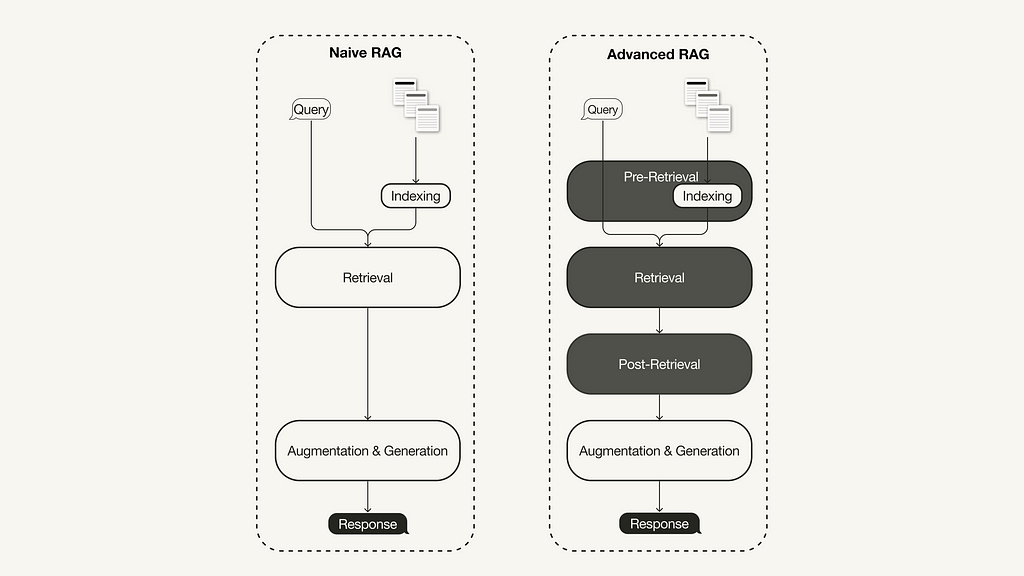
Difference between Naive and Advanced RAG (Image by the author, inspired by [1])
A recent survey on Retrieval-Augmented Generation (RAG) [1] summarized three recently evolved paradigms:
- Naive RAG,
- advanced RAG, and
- modular RAG.
The advanced RAG paradigm comprises of a set of techniques targeted at addressing known limitations of naive RAG. This article first discusses these techniques, which can be categorized into pre-retrieval, retrieval, and post-retrieval optimizations.
In the second half, you will learn how to implement a naive RAG pipeline using Llamaindex in Python, which will then be enhanced to an advanced RAG pipeline with a selection of the following advanced RAG techniques:
- Pre-retrieval optimization: Sentence window retrieval
- Retrieval optimization: Hybrid search
- Post-retrieval optimization: Re-ranking
This article focuses on the advanced RAG paradigm and its implementation. If you are unfamiliar with the fundamentals of RAG, you can catch up on it here:
Retrieval-Augmented Generation (RAG): From Theory to LangChain Implementation
What is Advanced RAG
With the recent advancements in the RAG domain, advanced RAG has evolved as a new paradigm with targeted enhancements to address some of the limitations of the naive RAG paradigm. As summarized in a recent survey [1], advanced RAG techniques can be categorized into pre-retrieval, retrieval, and post-retrieval optimizations.
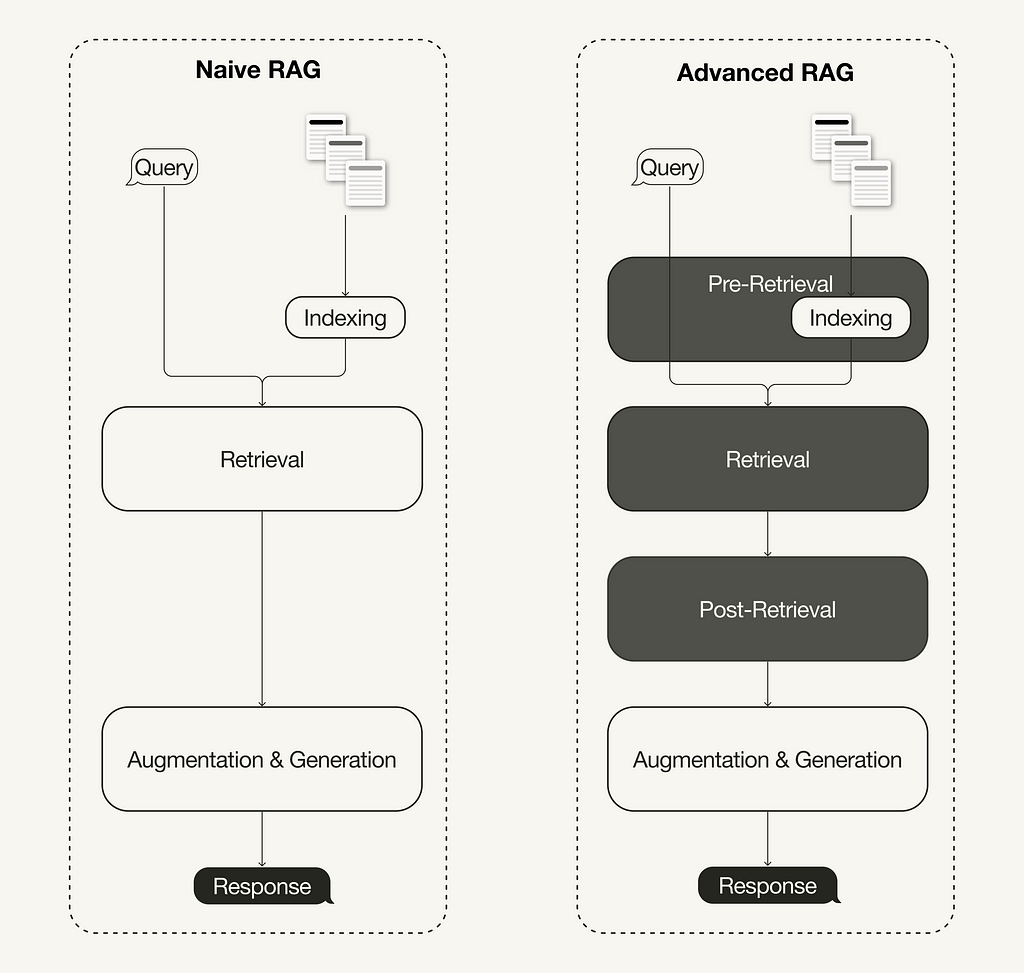
Difference between Naive and Advanced RAG (Image by the author, inspired by [1])
Pre-retrieval optimization
Pre-retrieval optimizations focus on data indexing optimizations as well as query optimizations. Data indexing optimization techniques aim to store the data in a way that helps you improve retrieval efficiency, such as [1]:
- Sliding window uses an overlap between chunks and is one of the simplest techniques.
- Enhancing data granularity applies data cleaning techniques, such as removing irrelevant information, confirming factual accuracy, updating outdated information, etc.
- Adding metadata, such as dates, purposes, or chapters, for filtering purposes.
- Optimizing index structures involves different strategies to index data, such as adjusting the chunk sizes or using multi-indexing strategies. One technique we will implement in this article is sentence window retrieval, which embeds single sentences for retrieval and replaces them with a larger text window at inference time.
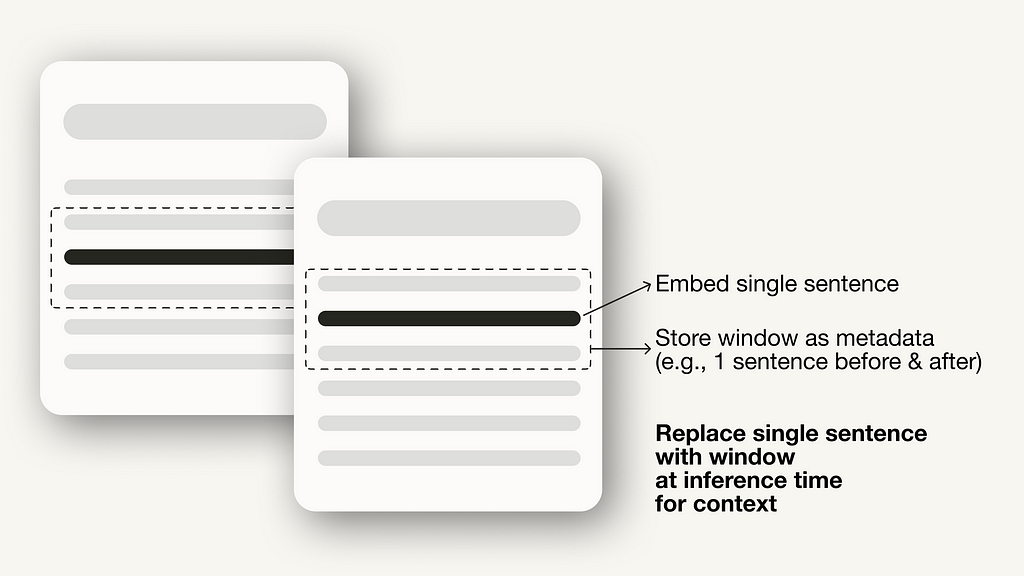
Sentence window retrieval
Additionally, pre-retrieval techniques aren’t limited to data indexing and can cover techniques at inference time, such as query routing, query rewriting, and query expansion.
Retrieval optimization
The retrieval stage aims to identify the most relevant context. Usually, the retrieval is based on vector search, which calculates the semantic similarity between the query and the indexed data. Thus, the majority of retrieval optimization techniques revolve around the embedding models [1]:
- Fine-tuning embedding models customizes embedding models to domain-specific contexts, especially for domains with evolving or rare terms. For example, BAAI/bge-small-en is a high-performance embedding model that can be fine-tuned (see Fine-tuning guide)
- Dynamic Embedding adapts to the context in which words are used, unlike static embedding, which uses a single vector for each word. For example, OpenAI’s embeddings-ada-02 is a sophisticated dynamic embedding model that captures contextual understanding. [1]
There are also other retrieval techniques besides vector search, such as hybrid search, which often refers to the concept of combining vector search with keyword-based search. This retrieval technique is beneficial if your retrieval requires exact keyword matches.
Improving Retrieval Performance in RAG Pipelines with Hybrid Search
Post-retrieval optimization
Additional processing of the retrieved context can help address issues such as exceeding the context window limit or introducing noise, thus hindering the focus on crucial information. Post-retrieval optimization techniques summarized in the RAG survey [1] are:
- Prompt compression reduces the overall prompt length by removing irrelevant and highlighting important context.
- Re-ranking uses machine learning models to recalculate the relevance scores of the retrieved contexts.
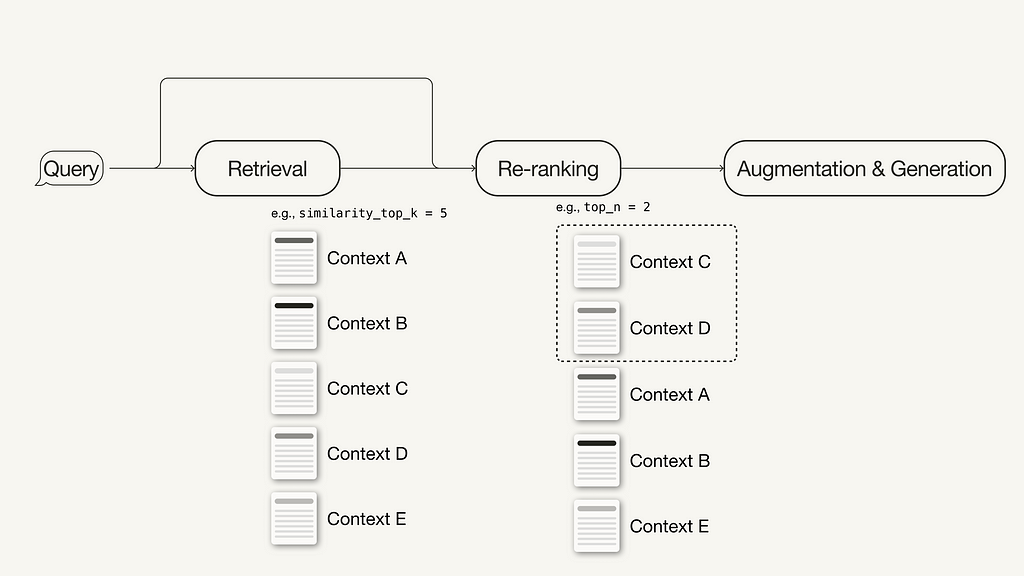
Re-ranking
For additional ideas on how to improve the performance of your RAG pipeline to make it production-ready, continue reading here:
A Guide on 12 Tuning Strategies for Production-Ready RAG Applications
Prerequisites
This section discusses the required packages and API keys to follow along in this article.
Required Packages
This article will guide you through implementing a naive and an advanced RAG pipeline using LlamaIndex in Python.
pip install llama-index
In this article, we will be using LlamaIndex v0.10. If you are upgrading from an older LlamaIndex version, you need to run the following commands to install and run LlamaIndex properly:
pip uninstall llama-index
pip install llama-index --upgrade --no-cache-dir --force-reinstall
LlamaIndex offers an option to store vector embeddings locally in JSON files for persistent storage, which is great for quickly prototyping an idea. However, we will use a vector database for persistent storage since advanced RAG techniques aim for production-ready applications.
Since we will need metadata storage and hybrid search capabilities in addition to storing the vector embeddings, we will use the open source vector database Weaviate (v3.26.2), which supports these features.
pip install weaviate-client llama-index-vector-stores-weaviate
API Keys
We will be using Weaviate embedded, which you can use for free without registering for an API key. However, this tutorial uses an embedding model and LLM from OpenAI, for which you will need an OpenAI API key. To obtain one, you need an OpenAI account and then “Create new secret key” under API keys.
Next, create a local .env file in your root directory and define your API keys in it:
OPENAI_API_KEY="<YOUR_OPENAI_API_KEY>"
Afterwards, you can load your API keys with the following code:
# !pip install python-dotenv
import os
from dotenv import load_dotenv,find_dotenv
load_dotenv(find_dotenv())
Implementing Naive RAG with LlamaIndex
This section discusses how to implement a naive RAG pipeline using LlamaIndex. You can find the entire naive RAG pipeline in this Jupyter Notebook. For the implementation using LangChain, you can continue in this article (naive RAG pipeline using LangChain).
Step 1: Define the embedding model and LLM
First, you can define an embedding model and LLM in a global settings object. Doing this means you don’t have to specify the models explicitly in the code again.
- Embedding model: used to generate vector embeddings for the document chunks and the query.
- LLM: used to generate an answer based on the user query and the relevant context.
from llama_index.embeddings.openai import OpenAIEmbedding
from llama_index.llms.openai import OpenAI
from llama_index.core.settings import Settings
Settings.llm = OpenAI(model="gpt-3.5-turbo", temperature=0.1)
Settings.embed_model = OpenAIEmbedding()
Step 2: Load data
Next, you will create a local directory named data in your root directory and download some example data from the LlamaIndex GitHub repository (MIT license).
!mkdir -p 'data'
!wget '<https://raw.githubusercontent.com/r...amples/data/paul_graham/paul_graham_essay.txt>' -O 'data/paul_graham_essay.txt'
Afterward, you can load the data for further processing:
from llama_index.core import SimpleDirectoryReader
# Load data
documents = SimpleDirectoryReader(
input_files=["./data/paul_graham_essay.txt"]
).load_data()
Step 3: Chunk documents into nodes
As the entire document is too large to fit into the context window of the LLM, you will need to partition it into smaller text chunks, which are called Nodes in LlamaIndex. You can parse the loaded documents into nodes using the SimpleNodeParser with a defined chunk size of 1024.
from llama_index.core.node_parser import SimpleNodeParser
node_parser = SimpleNodeParser.from_defaults(chunk_size=1024)
# Extract nodes from documents
nodes = node_parser.get_nodes_from_documents(documents)
Step 4: Build index
Next, you will build the index that stores all the external knowledge in Weaviate, an open source vector database.
First, you will need to connect to a Weaviate instance. In this case, we’re using Weaviate Embedded, which allows you to experiment in Notebooks for free without an API key. For a production-ready solution, deploying Weaviate yourself, e.g., via Docker or utilizing a managed service, is recommended.
import weaviate
# Connect to your Weaviate instance
client = weaviate.Client(
embedded_options=weaviate.embedded.EmbeddedOptions(),
)
Next, you will build a VectorStoreIndex from the Weaviate client to store your data in and interact with.
from llama_index.core import VectorStoreIndex, StorageContext
from llama_index.vector_stores.weaviate import WeaviateVectorStore
index_name = "MyExternalContext"
# Construct vector store
vector_store = WeaviateVectorStore(
weaviate_client = client,
index_name = index_name
)
# Set up the storage for the embeddings
storage_context = StorageContext.from_defaults(vector_store=vector_store)
# Setup the index
# build VectorStoreIndex that takes care of chunking documents
# and encoding chunks to embeddings for future retrieval
index = VectorStoreIndex(
nodes,
storage_context = storage_context,
)
Step 5: Setup query engine
Lastly, you will set up the index as the query engine.
# The QueryEngine class is equipped with the generator
# and facilitates the retrieval and generation steps
query_engine = index.as_query_engine()
Step 6: Run a naive RAG query on your data
Now, you can run a naive RAG query on your data, as shown below:
# Run your naive RAG query
response = query_engine.query(
"What happened at Interleaf?"
)
Implementing Advanced RAG with LlamaIndex
In this section, we will cover some simple adjustments you can make to turn the above naive RAG pipeline into an advanced one. This walkthrough will cover the following selection of advanced RAG techniques:
- Pre-retrieval optimization: Sentence window retrieval
- Retrieval optimization: Hybrid search
- Post-retrieval optimization: Re-ranking
As we will only cover the modifications here, you can find the full end-to-end advanced RAG pipeline in this Jupyter Notebook.
Indexing optimization example: Sentence window retrieval
For the sentence window retrieval technique, you need to make two adjustments: First, you must adjust how you store and post-process your data. Instead of the SimpleNodeParser, we will use the SentenceWindowNodeParser.
from llama_index.core.node_parser import SentenceWindowNodeParser
# create the sentence window node parser w/ default settings
node_parser = SentenceWindowNodeParser.from_defaults(
window_size=3,
window_metadata_key="window",
original_text_metadata_key="original_text",
)
The SentenceWindowNodeParser does two things:
- It separates the document into single sentences, which will be embedded.
- For each sentence, it creates a context window. If you specify a window_size = 3, the resulting window will be three sentences long, starting at the previous sentence of the embedded sentence and spanning the sentence after. The window will be stored as metadata.
During retrieval, the sentence that most closely matches the query is returned. After retrieval, you need to replace the sentence with the entire window from the metadata by defining a MetadataReplacementPostProcessor and using it in the list of node_postprocessors.
from llama_index.core.postprocessor import MetadataReplacementPostProcessor
# The target key defaults to `window` to match the node_parser's default
postproc = MetadataReplacementPostProcessor(
target_metadata_key="window"
)
...
query_engine = index.as_query_engine(
node_postprocessors = [postproc],
)
Retrieval optimization example: Hybrid search
Implementing a hybrid search in LlamaIndex is as easy as two parameter changes to the query_engine if the underlying vector database supports hybrid search queries. The alpha parameter specifies the weighting between vector search and keyword-based search, where alpha=0 means keyword-based search and alpha=1 means pure vector search.
query_engine = index.as_query_engine(
...,
vector_store_query_mode="hybrid",
alpha=0.5,
...
)
Post-retrieval optimization example: Re-ranking
Adding a reranker to your advanced RAG pipeline only takes three simple steps:
- First, define a reranker model. Here, we are using the BAAI/bge-reranker-basefrom Hugging Face.
- In the query engine, add the reranker model to the list of node_postprocessors.
- Increase the similarity_top_k in the query engine to retrieve more context passages, which can be reduced to top_n after reranking.
# !pip install torch sentence-transformers
from llama_index.core.postprocessor import SentenceTransformerRerank
# Define reranker model
rerank = SentenceTransformerRerank(
top_n = 2,
model = "BAAI/bge-reranker-base"
)
...
# Add reranker to query engine
query_engine = index.as_query_engine(
similarity_top_k = 6,
...,
node_postprocessors = [rerank],
...,
)
There are many more different techniques within the advanced RAG paradigm. If you are interested in further implementations, I recommend the following two resources:
Summary
This article covered the concept of advanced RAG, which covers a set of techniques to address the limitations of the naive RAG paradigm. After an overview of advanced RAG techniques, which can be categorized into pre-retrieval, retrieval, and post-retrieval techniques, this article implemented a naive and advanced RAG pipeline using LlamaIndex for orchestration.
The RAG pipeline components were language models from OpenAI, a reranker model from BAAI hosted on Hugging Face, and a Weaviate vector database.
We implemented the following selection of techniques using LlamaIndex in Python:
- Pre-retrieval optimization: Sentence window retrieval
- Retrieval optimization: Hybrid search
- Post-retrieval optimization: Re-ranking
You can find the Jupyter Notebooks containing the full end-to-end pipelines here:
Enjoyed This Story?
Subscribe for free to get notified when I publish a new story.
Get an email whenever Leonie Monigatti publishes.
Find me on LinkedIn, Twitter, and Kaggle!
Disclaimer
I am a Developer Advocate at Weaviate at the time of this writing.
References
Literature
[1] Gao, Y., Xiong, Y., Gao, X., Jia, K., Pan, J., Bi, Y., … & Wang, H. (2023). Retrieval-augmented generation for large language models: A survey. arXiv preprint arXiv:2312.10997.
Images
If not otherwise stated, all images are created by the author.
Advanced Retrieval-Augmented Generation: From Theory to LlamaIndex Implementation was originally published in Towards Data Science on Medium, where people are continuing the conversation by highlighting and responding to this story.